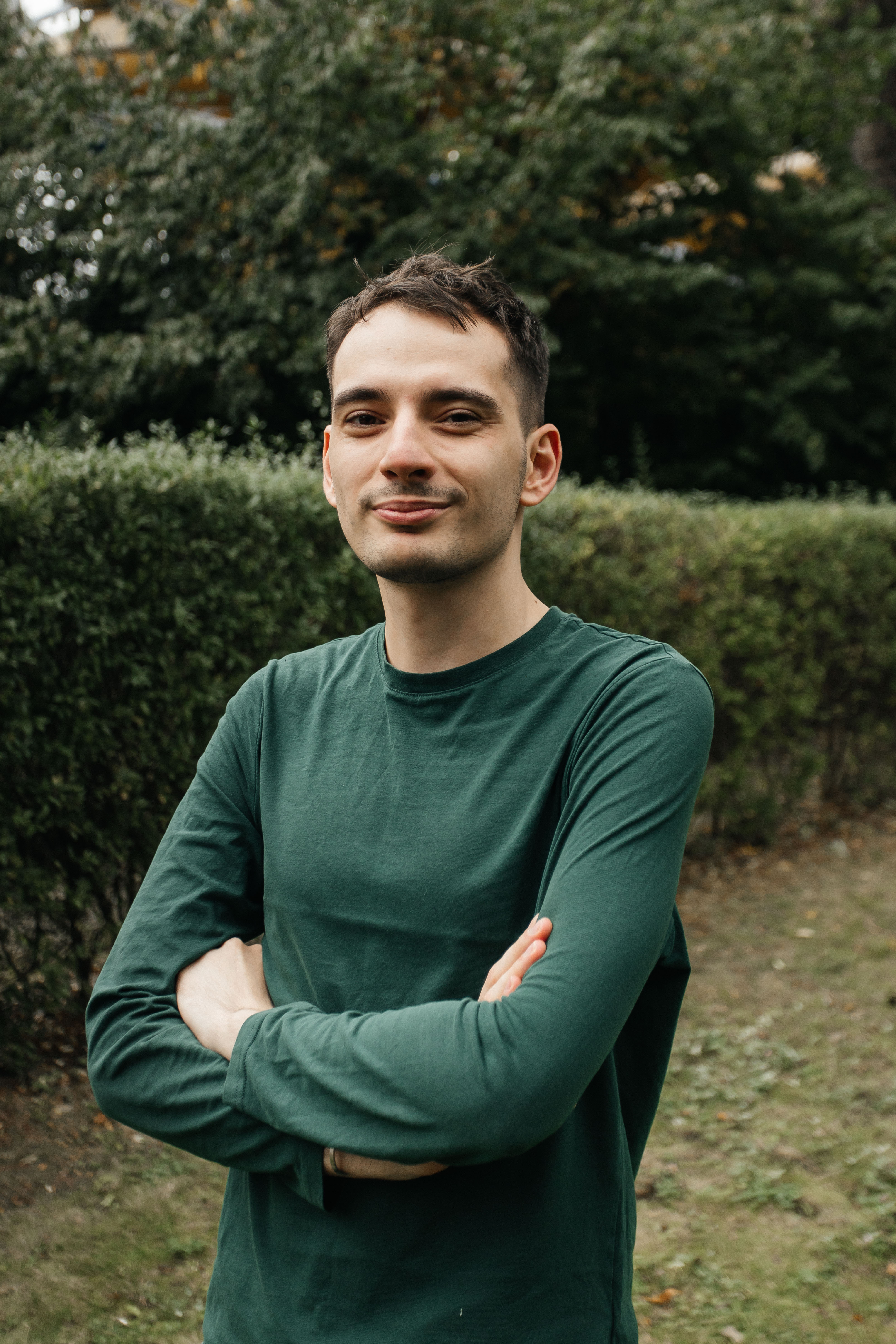
I am currently a PhD student at CERGE-EI. I specialize in econometrics, with a focus on instrumental variables estimation, dimension asymptotics, and causal inference.
My supervisor is Stanislav Anatolyev. In Spring 2025, I am visiting the Department of Economics at MIT, where my host is Anna Mikusheva.
I have received my BA from HSE and UPF. During my PhD, I have visited CEMFI where Dmitry Arkhangelsky was my host.